Agentic AI: technology with a can-do attitude
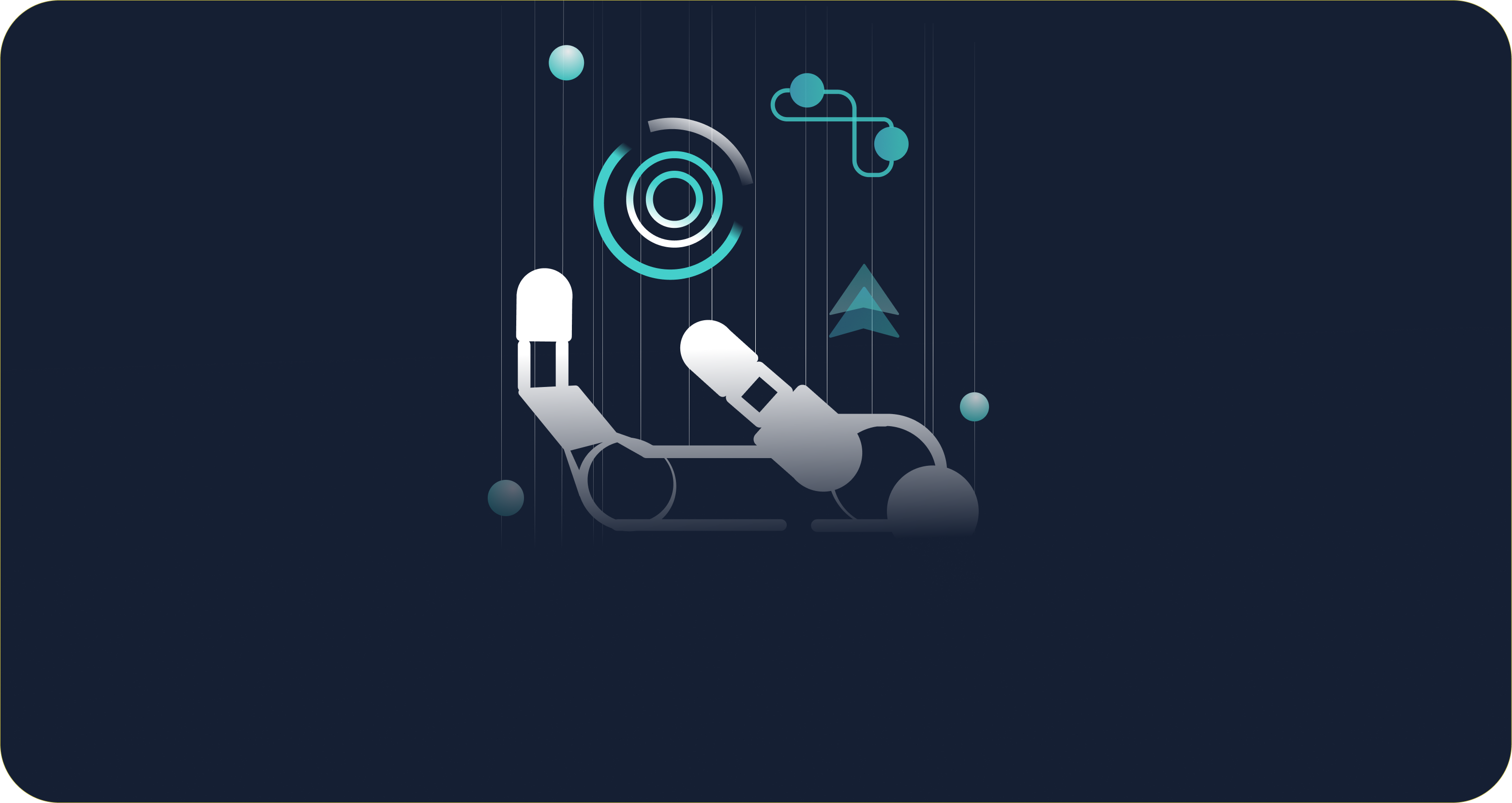
Technology with a can-do attitude
Have you ever been in charge of training a new hire? Explaining both how and why certain tasks need to be done takes time—and time is often in short supply. When your to-do list keeps growing, the last thing you want to hear is, “Okay, what do I do next?” Wouldn’t it be easier if you could simply share the goal and let them figure out the steps from there?
The latter scenario is closer to reality than you might think with agentic AI. This latest AI advancement will take productivity and efficiency to the next level by reducing the need for human intervention in complex tasks. Think of it as a goal-orientated AI that can think, act, and learn to complete tasks using the tools at its disposal.
It should come as no surprise that if agentic AI can live up to its potential, an estimated “33% of enterprise software applications” will include agentic AI by 2028.
For the financial services industry, agentic AI can relieve the pressure of navigating complex issues like managing risk and adapting to market changes in real time. However, in these early days, questions about regulatory compliance and quality assurance need to be addressed. In the meantime, it’s best to start with a foundational understanding of this technology to inform an FI’s agentic AI strategy down the road.
What is agentic AI?
Agentic AI refers to a comprehensive network of AI systems with the ability to assess a situation, plan a course of action, and carry out tasks to accomplish a goal or objective. There are many characteristics that define agentic AI, but three main ones include:
Self-Direction
Once a goal has been set, it can plan each step and task based on the data and tools it’s provided without needing a human to explain the process.
Autonomous decision-making
Once the right steps have been determined, they can be carried out by the AI agents to meet the goal.
Real-time adaptation and learning
It can continuously monitor performance and learn from human interactions and feedback to refine its approach in real time.
In other words, agentic AI is like a new hire who never has to ask what to do next at each step. It is self-sufficient and will use the tools and information provided to accomplish the set goals.
Agentic AI compared to GenAI and machine learning
To understand the key distinction between these types of AI, let’s review how supervised machine learning models and GenAI can be integrated into a lending operation.
Machine learning (ML) models in lending
• AI-automated underwriting: Supervised machine learning models can be trained on millions of data points to accurately predict if borrowers will default on a loan.
• Fraud detection: These models can also be used to detect subtle signals of first and third-party fraud—from synthetic identities to inconsistencies with reported income and employment indicators.
Generative AI (GenAI) in lending
GenAI tools can generate lending insights by instantly analyzing data such as portfolio performance, credit policies, and economic and industry reporting with just a few prompts. Uncovering these strategic insights faster allows FI to make more informed and proactive decisions.
In these scenarios, GenAI and machine learning models play a crucial role in making lending operations more efficient. Both AI-automated underwriting and fraud detection can run processes autonomously, but often, there is still human intervention involved when, for example, a lending strategy changes and rules need to be updated to meet new goals.
With GenAI, the analyst or business leader saves time on the data analysis process, but still has to verify that the insights or recommendations are compliant before implementing them.
Potential benefits of agentic AI for financial services
Agentic AI’s ability to act with greater independence could help streamline lending operations with stronger, real-time results. These use cases for financial services are just scratching the surface of what agentic AI has the potential to do.
Streamline customer upsells
A customer-facing AI agent could proactively recommend credit card offers or refinancing opportunities when a borrower’s qualifications improve. The AI could then initiate outreach and begin the application process by gathering basic information and collecting the necessary documents from the borrower.
Round-the-clock fraud prevention
Instead of just flagging suspicious applications, a fraud agent could preemptively block high-risk lending scenarios before fraud occurs. It can also adjust risk thresholds, log fraud signals in a database, and even test new countermeasures 24/7.
Automatic loan risk balancing
An AI lending agent could autonomously review and rebalance an FI’s loan portfolio by analyzing shifts in risk and interest rates. It could identify overexposure to certain loan types (e.g. auto loans, mortgages, credit cards) and take the necessary actions to make the adjustments.
Challenges with agentic AI
An AI system that can think and act independently has drawbacks, especially for highly regulated industries like lending and financial services. Potential hurdles for FIs include minimizing the use of low-quality data, ensuring regu
latory guidelines are followed, and, most importantly, allocating the necessary resources to build and maintain an agentic AI platform.
Low data quality
AI is only as good as the data it’s trained on. If the data is biased, incomplete, or outdated, it will limit the agentic system’s ability to carry out the necessary actions to achieve its objective. Whether it’s a supervised machine learning underwriting model or agentic AI, using high-quality, ethically sourced data is the best place to start to ensure accuracy within a lending operation.
Operating without sufficient regulatory guardrails
Without strict regulatory oversight and ethical safeguards, agentic AI could make decisions that violate fair lending laws and bring an FI into noncompliance. Stringent guardrails are a must in an industry where a lack of transparency and discriminatory practices have high financial and reputational consequences. While training AI to adhere to policies is possible, there is little room for error with agentic AI operating independently.
Implementation and quality assurance
In these early days, few off-the-shelf agentic AI solutions exist. Getting agentic AI up and running requires significant work to build the necessary infrastructure and integrate legacy systems, followed by rigorous testing of the outputs. Even if the data sets are high quality, a poorly configured agentic AI system that can’t execute tasks accurately will create more work for teams across the lending organization to manage. For many FIs, agentic AI may not be worth the ROI until the technology has had time to mature and become more accessible.
Staying ahead of what’s next with AI
Even though the potential of agentic AI hasn’t been fully realized yet, that doesn’t mean lenders should hold out on adopting AI altogether. In fact, GenAI lending intelligence tools like LuLu already incorporate some self-guided agentic qualities. Running accurate business-critical tasks like policy simulations and in-depth peer analysis comes naturally to a GenAI tool built for the lending industry and only requires a few prompts to complete.
For the FIs with their sights set on adopting agentic AI, walking before you run is important. Investigating GenAI and other machine learning solutions like LuLu, AI-automated underwriting, and fraud detection models today will put you ahead of the rest until agentic AI becomes widely accessible.