CFPB calls for use of AI, break with traditional scoring methods
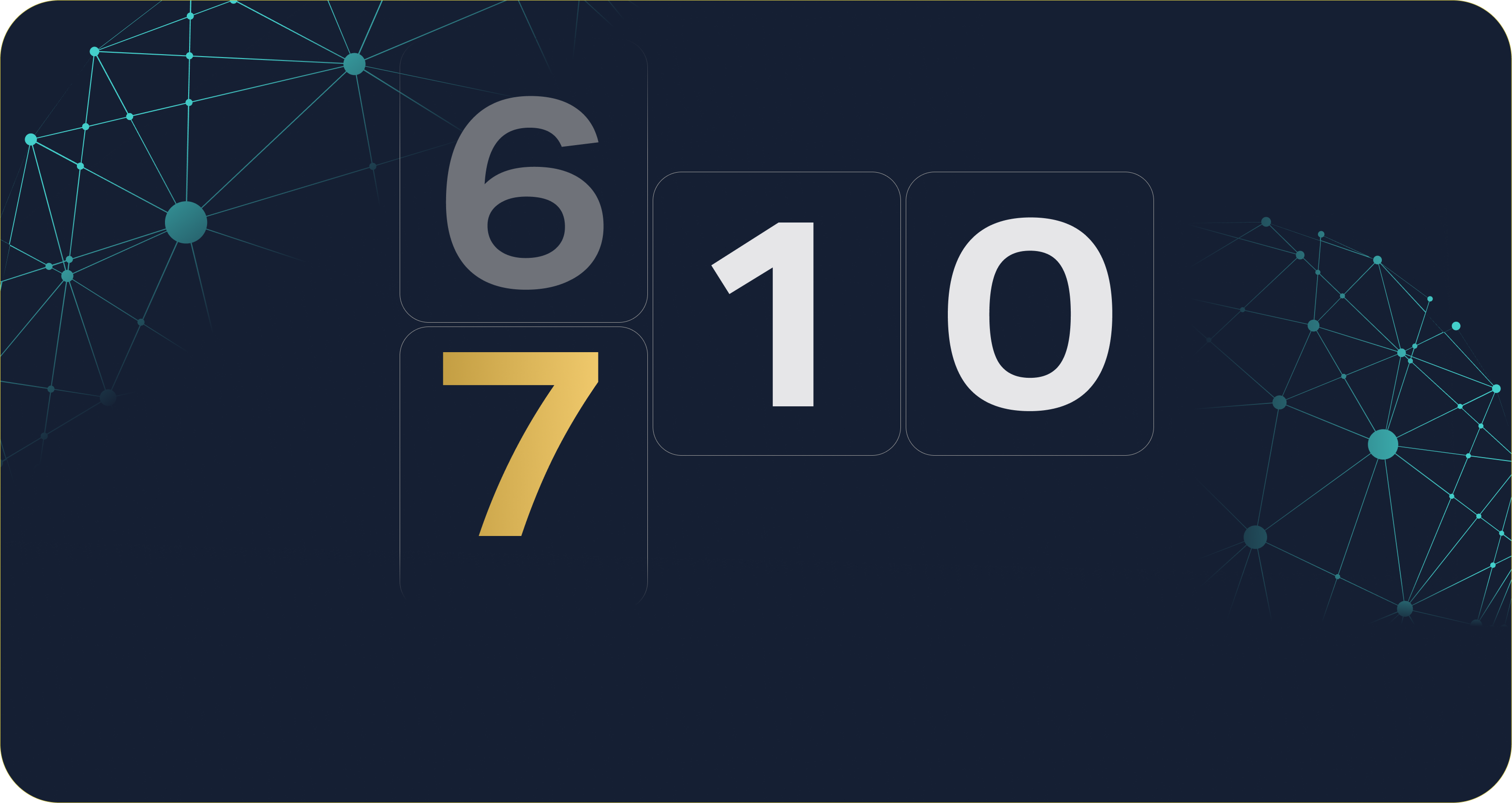
The need for purpose-built AI to modernize credit scoring models
When the status quo continues to be detrimental to businesses and consumers, engaging with government agencies on how to effect change is critical to moving forward. The Consumer Financial Protection Bureau’s Director Rohit Chopra spoke about traditional credit scoring models in November, and given Zest AI’s ongoing advocacy for a level credit-scoring playing field I was encouraged by his call to action to look to AI as a solution.
Director Chopra spoke on a number of important issues, but specifically that traditional credit scoring needlessly excludes Americans from getting assessed for credit and increases costs for financial institutions without commensurate benefit. He noted that government policies must change so that market participants can confidently explore AI/ML to improve credit scoring accuracy. Zest AI has highlighted this topic repeatedly (see here, here, and here, for example).
During his speech at the recent AI Symposium hosted by FinRegLab, he encouraged government agencies to rethink longstanding policies that steer lenders toward traditional credit scoring methods. American Banker quoted Director Chopra:
“…[G]oing forward I think we have to adjust government policies that push the market toward the use of traditional credit scores, and create the conditions for a meaningful, helpful and transparent use of AI.” AB went on to report that Chopra said the ability of consumer loans to be securitized via the FICO score has created a challenge for banks and other financial firms. “Lenders report to the CFPB that credit scores are really just not predictive enough anymore,” Chopra said. “To stay competitive, major lenders build their own proprietary scorecards to evaluate applications, and many would like to abandon standardized scores if they could, if not for that [Fannie and Freddie] liquidity premium they get from it.”
Over several years Zest AI has called on federal and state policymakers to support new credit scoring systems that improve on legacy approaches. We believe that strong guidelines for algorithmic credit scoring transparency can create a level playing field and more opportunities for all Americans to access equitable lending.
The path toward inclusion with purpose-built AI
Chopra remarked that using a better model built using AI that moved lenders away from the traditional credit score would promote inclusion and competition. He suggested that the government work with the private sector to develop a new open-source model, with inner workings well understood by all concerned and that could be used on a cooperative basis.
There is much to applaud in this suggestion. It is true that relying on widely adopted open-source software (OSS) packages for building predictive models (classification or regression) can create consistency. When different financial institutions, agencies, and vendors are using the same underlying base packages, it prevents vendor lock-in and increases competition.
However, the ability to address more precise issues and serve diverse populations requires customization. OSS must support a broad community, which can hamper its ability to tackle institution-specific problems and protect consumers. For example, to date, OSS cannot generate fast yet accurate adverse action reason codes in production. This is a critical requirement for lending that takes time, expertise, and resources to develop. While OSS provides a strong foundation, investment by specialized firms in proprietary refinements and enhancements is often necessary.
Additionally, reliance on one national OSS model is not optimal for financial inclusion. There is extremely effective technology to customize models available right now even if they are built on open-source packages. Open access to robust, representative, compliant data is critical to inclusive models, and this data is often unique to lending areas and institutions. In our experience with our credit union and bank clients, models purpose-built for the institution work best to maximize benefits and understand risk. The institution has visibility into the data used in the purpose-built AI/ML model, its build, and ongoing insight into the model’s operation for monitoring and audit purposes. Customization allows for the creation of models that are both effective and equitable, while maintaining the familiar structure of OSS packages helps make them easier to explain and deploy.
As a final observation, policymakers and AI stakeholders are increasingly interested in mandating that decisioning models be made available as open-source software. This is perhaps to codify open-source as a hallmark of transparency since OSS belongs to the commons rather than to any one owner.
Open-source should not be understood as a necessary pre-requisite to achieve transparency into models, or as ‘better’ than proprietary technology. AI/ML models built with proprietary intellectual property like the hundreds of models developed by Zest AI can provide visibility into all of the data variables used to train the model as well as their interactions. Zest scientists, engineers, and attorneys ensure that Zest AI models generate explainable and accurate adverse action reason codes, one of many requirements mandatory under existing law regardless of the tool used.
As FIs, fintechs, and regulators move forward in policy discussions around standards for AI in the financial services industry, we will continue to advocate for transparency and the ability to create a level playing field for lenders and the communities they serve.
Yolanda D. McGill. Esq. – VP, Policy and Government Affairs
Yolanda is Zest AI’s Head of Policy and Government Affairs. She is a practicing attorney with more than 20 years of experience in financial services. Since 2003, she has worked for mission-driven organizations such as the Lawyers’ Committee for Civil Rights Under Law and the Consumer Financial Protection Bureau, providing highly technical legal expertise with a personable approach to complex topics.