What lenders should know about behavioral fraud detection
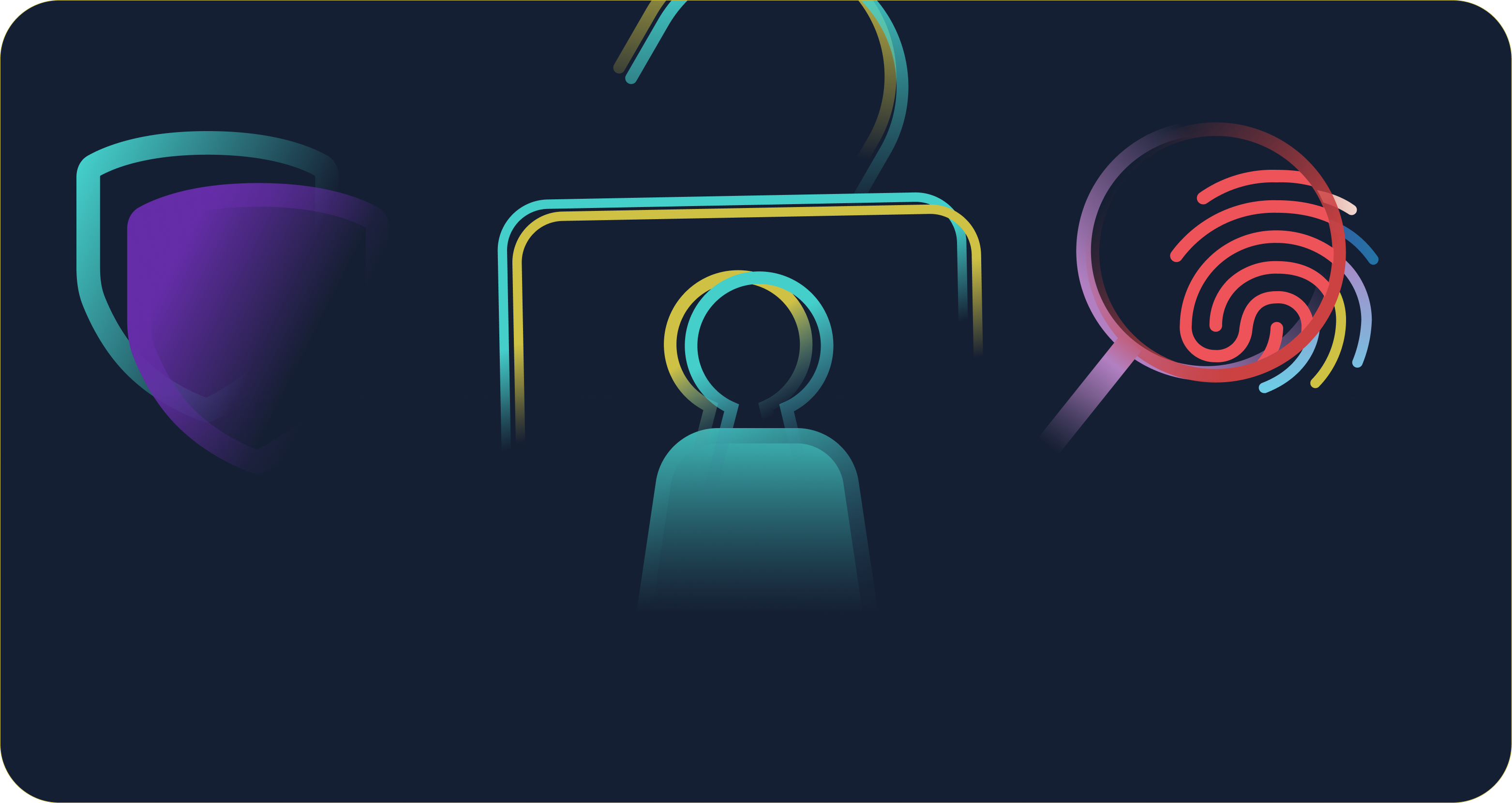
Why behavioral fraud detection
Picture this: An underwriter reviews an application that seems perfectly fine on the surface. The credit score is strong, and the income looks accurate, with no obvious red flags in the employment history. With everything appearing legitimate, the loan is approved. Months later, the other shoe drops. After numerous failed attempts to contact the applicant, the once-promising borrower disappeared, leaving behind a charged-off loan and thousands of dollars in losses.
Unfortunately, scenarios similar to this are increasing across the country with many types of lenders. Fraud is rapidly evolving, and financial institutions (FIs) are feeling the pressure. According to a recent industry study, the percentage of synthetic identities among accounts opened reached record highs in 2024.
As generative AI (GenAI) tools become more accessible, fraudsters find sophisticated ways to dupe lenders and exploit process vulnerabilities. It shouldn’t come as a shock that the number of fraud attempts—and associated losses—is expected to grow in the coming years.
Recognizing the signals of bad actors is the key to combating this rise in fraud. This is why banks and credit unions need to have a strong understanding of behavioral analytics, holistic fraud detection, and how instant insights can help catch fraud before it happens.
How does behavioral fraud detection work?
Behavioral fraud detection is the practice of analyzing patterns, actions, and anomalies in a borrower’s behavior to uncover signs of fraudulent activity.
A fraudster’s tactics are often subtle, intending to exploit the limitations of an FI’s application or account onboarding process. Some incidents are one-off attempts, while others are part of a complex web of schemes that impact multiple institutions.
To uncover this type of fraud, institutions primarily use manual reviews and traditional detection methods. However, sophisticated machine learning models are one of the best ways FIs have to identify subtle signals and patterns over time that can paint a larger picture of who a borrower really is. Fraudsters may use various tactics like inflating personal details, falsifying documents, or even creating synthetic identities, but each of these leaves clues that can be spotted with advanced behavioral analytics.
Behavioral fraud detection can help identify a broad set of fraud types, including first-party and malicious intent, to third-party including synthetic fraud.
What is first-party fraud?
First-party fraud is when an individual uses their own identity but often manipulates the details (i.e., income or job history) to have a lower risk profile for increased credit credit. Below are some examples of what a behavioral fraud model could detect.
Bust out fraud
In a bust out fraud situation, a fraudster applies for a credit card, and after a period of normal activity, the account holder maxes out the card with no intention of repaying the balance.
Sleeper fraud
With sleeper fraud, the fraudster follows the same method of bust out fraud but with a longer period of “normal” activity. The intent is to demonstrate good borrower behavior to secure more credit line offers. Then, after months or even years, the fraudster will abscond with the funds and never repay their loan balances.
First-party fraud indicators can include:
- An applicant has multiple unused credit cards but is applying for another card
- Inconsistencies between stated income and outside data sources
- Application details that seem “too good to be true”
- A history of credit line increases followed by sudden defaults
- Numerous applications with slightly altered personal details (i.e., home address)
First-party fraudsters might not be considered the most sophisticated of bad actors, but their tactics can still be challenging for FIs to detect.
What is third-party fraud?
Unlike first-party fraud, this type occurs when a fraudster uses stolen information to apply for a loan, often as the outcome of identity theft. Fraudsters obtain personally identifiable information (PII), like Social Security numbers or home addresses, usually through a data breach, and then use that information to submit a loan application.
Synthetic identify fraud
Synthetic identity fraud happens when a fraudster creates a new identity using components of real identifying information and fake or synthetic information. They then use this new synthetic identity to apply for a loan. If approved, the funds are routed to the fraudster’s bank account. By the time the fraud is detected, the fraudster is long gone.
Third-party fraud indicators include:
- Discrepancies with addresses or phone numbers compared to public records
- The IP address doesn’t match the stated address on the application
- After a period of inactivity, multiple new tradelines were recently opened
Third-party fraud is especially damaging because it impacts both the financial institution and the victim(s) whose identity is compromised. The ability to detect and prevent these schemes protects FIs from financial loss and builds trust with borrowers by safeguarding their identities.
Where origination fraud detection methods fall short
Fraudsters are constantly evolving tactics, but legacy fraud solutions have been slower to adapt to the nuances of sophisticated fraud. Solutions that provide traditional identity or income verifications are only components of a holistic approach. With the frequency of false positives and opaque outputs, lenders have decreased confidence and increased reliance on manual reviews.
Strong behavioral models can detect fraudulent details that aren’t as straightforward. They provide a more holistic approach to origination fraud detection by providing fast, accurate, and actionable holistic fraud insights.
Lenders who implement a holistic approach to fraud detection will have a significant advantage over lenders who continue relying only on basic verification solutions.
AI is changing how FIs fight fraud
The hope for FIs future-proofing their fraud detection lies in using the power of AI advancements like sophisticated machine learning (ML) models. Even though fraudsters constantly evolve their strategies, AI can adapt to them.
ML offers a level of accuracy, speed, and adaptability that traditional methods simply cannot match. These ML models can be retrained on new data, allowing them to recognize emerging fraud patterns and respond proactively. By analyzing behavioral patterns and detecting subtle discrepancies in borrower data, banks, and credit unions can stay ahead of even the most sophisticated fraud schemes.