Mythbusting common AI misconceptions in the credit union industry
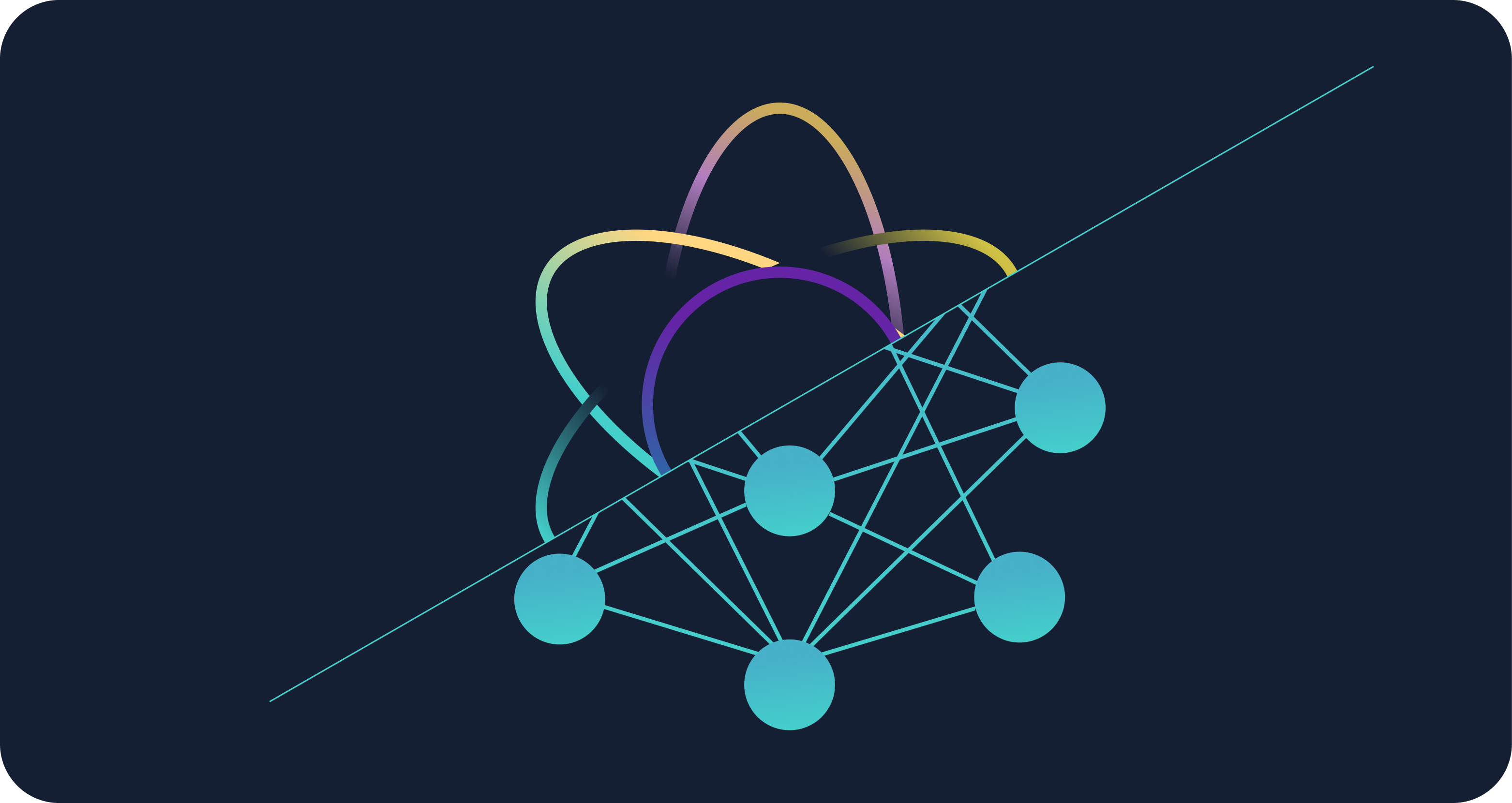
At this point, the statement “AI is booming” is as obvious as “water is wet” or “the sky is blue.” Everywhere you look, companies, organizations, and individuals are adopting the latest available AI tool or app to do, well… anything! AI is such a broad term that it can mean implementing a chatbot, automating administrative work, creating fantastical images, predicting consumer behavior, and so much more.
This buzz has created a race amongst many organizations to start adopting AI, but with varying degrees of understanding and success. As a result, the demand for AI in every industry, including credit unions, has skyrocketed. From our latest Cornerstone Advisors report, 54% of community-based financial institutions plan on investing over $100,000 in AI within the next 3 years.
As we know, the internet can blur the lines between fact and fiction— AI is no exception. Let’s dig into some of the common misconceptions about AI, and what it really means for your credit union today.
Expectation: AI is a brand new technology that’s only been used in the last few years. It’s too immature of an invention to safely adopt.
Reality: AI and machine learning models have been around since the 1950’s.
In 1950, mathematician Alan Turing created the “Turing Test” to determine if computers could be as intelligent as human beings. Ever since, the field of computer science has grown and developed programs for solving problems, ranging anywhere from playing chess to understanding human language. Over time, these techniques have evolved to use even more information and solve more complex issues, like predicting borrowers’ behavior patterns.
In more recent decades, we have incorporated AI into our lives with technology like email spam filters, online shopping recommendations, GPS apps, and voice assistants like Siri and Alexa.
Expectation: All AI is just like Chat GPT.
Reality: While ChatGPT is a popular AI tool, not all AI technology works the same way.
ChatGPT is a generative AI tool that sources large amounts of data to create human-like responses. It uses a large language model (LLM) to learn relationships between words and their meanings. Generative AI models can be broad like ChatGPT which can pull data from across the internet, or trained to specialize in one area. For example, this app helps detect skin cancer. When using specific data sources from your organization, generative AI can help with anything from customer service to strategic guidance. It can access and organize multiple data sources without having to manually build dashboards or reports, providing insights in a clean, seamless manner.
While generative AI is useful, it’s only one type of AI tool. On the other hand, machine learning models focus on identifying patterns in data and making accurate predictions. For instance, it can more accurately predict credit risk by diving deep into a credit report and finding more correlations and patterns between thousands of variables to determine the likelihood of default. It can also detect fraud, flagging things like misreported income or identity theft using sophisticated algorithms.
There are many types of AI today. For lenders, the two most common are:
- Machine learning (ML). Used for things like credit underwriting and fraud detection, machine learning uses algorithms to more accurately predict specific outcomes, such as how risky an applicant is. These AI models are “locked” and do not learn on their own, allowing for all decisions and data to be transparent and consistent.
- Generative AI (GenAI). As described above, generative AI can create new content from various data sources, versus prescribed outputs with ML, and can keep “learning” based on new inputs. Used for functions like chatbots and intelligence reporting, GenAI can create a lot of efficiencies but is not created to make decisions on its own, like ML as it requires human due diligence.
Expectation: Implementing AI in your organization will lead to a robot takeover.
Reality: Incorporating AI strategically, and with the right partners will strengthen your organization.
Ok, maybe “robot takeover” was a bit dramatic. Regardless, several industries (not just financial services) have a lot of fear surrounding how AI will impact their jobs. In the financial services industry, AI models have the potential to significantly decrease time spent on administrative tasks, freeing up underwriters and credit analysts to tackle more complex issues.
For instance, implementing AI-automated underwriting using machine learning models can allow credit unions to automate up to 80% of all consumer credit decisions. That still leaves 20% of applications that will require a human touch. Usually, these applications are from members who need more time and support.
Instead of handling the bulk of applications that machine learning algorithms can easily and accurately decision, underwriters have time to dig deep on the loans that need human oversight, hold more meaningful conversations, and offer advice and support that will set them up for success.
For analysts and leaders, having access to generative AI tools that can quickly synthesize large amounts of data is a game changer for making more proactive and informed decisions. You’ll still need experts to interpret and make decisions based on the information, but now you can do it much faster and more frequently.
Expectation: AI can get you in trouble with regulators.
Reality: AI can allow you to be more compliant and less biased.
It is true that not all AI algorithms are created equal, and some can exacerbate biased decisioning. This poses a real risk for lenders, who need reliable credit decisioning models that will truly give everyone a fair shot.
That’s why it’s critical that AI decisioning models be fully transparent, documented, and undergo thorough fair lending tests. This includes a search for the least discriminatory model, or the model that is proven to mitigate disparate impact for protected class borrowers.
When these models are properly tested to lessen disparity, they can increase approvals and improve lending outcomes for protected classes without increasing risk. For example, Verity Credit Union has been able to increase approvals by 271% for individuals aged 62+, 177% for Black Americans, 375% for Asian Pacific Islanders, 194% for women, and 158% for Latinos through the use of AI-automated underwriting.
Expectation: AI is unstable and untrustworthy.
Reality: The right technology partners will “lock” their AI.
Locked AI refers to machine learning models that are trained on specific data sets and do not learn on their own. This allows outputs (like lending decisions) to be more easily monitored and produce consistent results. In contrast, an unlocked model poses risks because its results can’t be easily explained or monitored. It’s constantly adding and reacting to new variables, obscuring your ability to see how accurate its lending decisions are.
Machine learning models that are locked and supervised–meaning that they are fully explainable and will not become a “black box” of predictions–will be able to produce robust documentation that can be used to satisfy examiners.
It’s critical that lenders have robust documentation for their AI models, which will include the data used, fairness testing, and all details necessary for a compliant, transparent model. Locked AI models will produce consistent, accurate results that can be thoroughly documented.
Even when using a generative AI tool, it should still be trained on a specific data set and given a specific use case. Generative AI should never make credit decisions for you, but it can help you access and organize information that will help formulate your lending strategy.
Expectation: AI is just for big FIs
Reality: AI is accessible to all types of institutions, no matter how big or small
It’s no secret that big banks are using AI, but credit unions of all sizes have been pioneering the use of AI technology for years. AI gives community-based lenders a more level playing field against larger institutions—not only do they have the same, powerful machine learning technology at their fingertips, but they also have a local touch that makes for a more personalized experience. Tailored AI models add value to lenders serving niche markets, including specific regions, communities, or professions.
Credit unions that process only around 100 loan applications a year, and those under $2M in assets, have been using the same AI technology for underwriting as those processing 100s of thousands of applications, and far larger than $1B in assets.
Expectation: AI is magic and spells.
Reality: It’s just math.
At Zest AI, we like to say, “the key is more data and better math.” AI can do incredible things, but it can be even stronger when used with intention and understanding.
When you look under the hood of any technology (especially powerful AI models), you’ll discover human beings behind the scenes, putting their heads together to figure out the best way to tackle an issue. Whether it’s programming a computer to play checkers or developing a more accurate way to assess credit risk, it’s the diverse perspectives and expertise of people that drive innovation.
High-performance machines require high-caliber data scientists. The success isn’t due to a mysterious algorithm or a black box; it’s the result of a determined, problem-solving team. While AI may seem like magic, it’s the thoughtful application of data science and human ingenuity that truly brings its potential to life.
________________________________________________
Regarding the artwork…
Expectation: We spent hundreds of hours drawing these illustrative comics.
Reality: AI generated these crazy images!
Hope you found them as fun as we did!